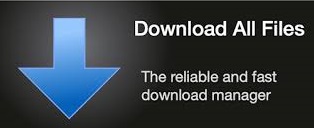
Similarly, if a selected node has no edge connection to any other terms in the set, it will not be displayed or included in the graph structure. The feature is useful for understanding terms in larger more complex graphs or for terms which have acronyms and/or domain jargon.ġA bit about the logic: if there are no dependencies among the selected terms, nothing will be displayed. The nodes are not "clickable objects" per se such as in a d3 model, so the layout matching is somewhat inexact, but it is close enough to be functional. This works by mapping click locations in the client side graph layout to the server side layout and identifying the nodes. Clicking on nodes will produce any existing annotations or definitions of the term in the network graph (via sparql query of the ontology) and display them just below the graph itself. The network toplogy graph is also interactive.

#HUGIN BAYESIAN DOWNLOAD#
Fortunately, there are ways to save your work as you go (see Download Panel).
#HUGIN BAYESIAN SOFTWARE#
As previously mentioned, because the software is reactive, changes in inputs (ontology choice, selected nodes, dependency slider, etc) will automatically result in recomputation and redrawing of the network display and any other dependnent factors on other tab panels. With respect to levels of evidence, one can use this slider to adjust or examine the strength of the overall network. Networks are updated in real-time as this value is changed. If no specific strength is provided, all arcs are considered level 1 strength. For an ontology that contains a layered dependency such as this, the "Dependency Slider" can be changed to exclude nodes below the selected level.
#HUGIN BAYESIAN TRIAL#
The "Evidence" term used comes from a medical context and represents a ranking system used to describe the strength of the results measured in a clinical trial or research study, though this value could represent some other type of dependency more generally. The "Mean Evidence" metric is the mean value of the set of dependency arcs strengths. If the dependency properties in the ontology have numeric tags specifying the strength of dependency, ("dependsOn3", for example) then some statistical metrics describing the network (nodes and edges) will be computed and displayed above the graph. If dependencies among the selected concepts exist, then the network graph belonging to this set of concepts will be immediately computed and displayed in this panel 1. The checkbox group is also selectable, such that network nodes can be removed and added from the graph without re-navigating the folder tree. Some ontologies are too large to view easily in a folder display, so this list is here to keep track of all the things you have selected so far. Selection of a concept or category of concepts will add it and any subconcepts to the checkbox list just to the right of the folder tree. By holding the 'ctrl' key you can select multiple concepts. With an ontology loaded, you can now select among the various concepts shown in the folder tree. This folder tree structure is an instance of jstree and is therefore interactive and selectable. Once an ontology is selected, the software will automatically read the class-subclass hierarchy and recreate it in the main sidebar panel as a folder tree.

These ontologies contains object properties that define the dependencies between classes. A simple ontology ("testontology.owl") is available for experimenting with software features. If you upload an ontology it will appear in the selectable list of available ontologies. Here, you can select a preloaded ontology or load one of your own. The main sidebar panel has options to do both. In order to use this tool, you must select or upload an ontology. The interface is also interactive, thus, changes in concept selection, dependency level, and other parameters result in real-time updating of the graphics and other reactive features. This eases the development of Bayesian networks in that the user need not reconstruct a network from scratch for every use case, nor search the literature or interview domain experts to establish an appropriate network structure. When the user selects a set of concepts of interest, the tool will automatically create the network nodes and arcs in a graph object and display the structure of the network, among other things (described in more detail below). The domain ontology specifies what concepts are dependent on others, so it is not neccessary to have a priori knowledge of what they are. The primary use of this software is to semi-automate the construction of dependency networks (Bayesian networks) from a domain ontology knowledge base.
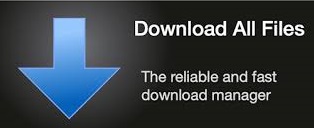